What Is Data Wrangling?
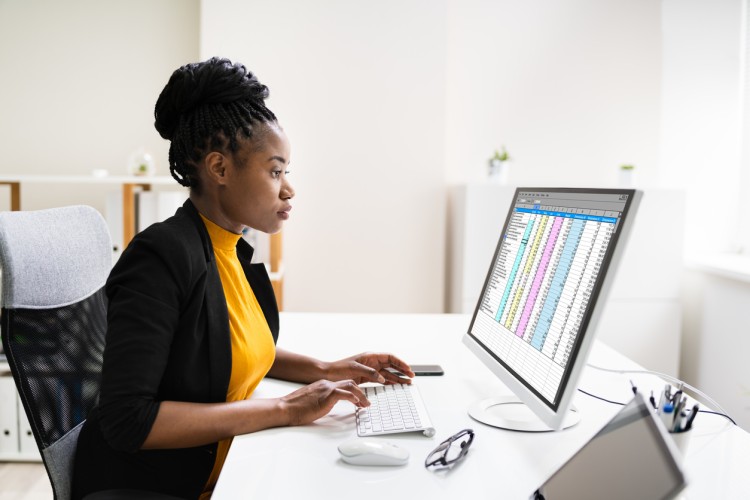
The world generated an estimated 2.5 quintillion bytes of data daily in 2020, and experts project that number will grow to 436 daily exabytes by 2025. In this era of big data, growing numbers of businesses across a wide range of industries rely on data wrangling professionals to help them sort, clean, and restructure their data into analysis-friendly formats.
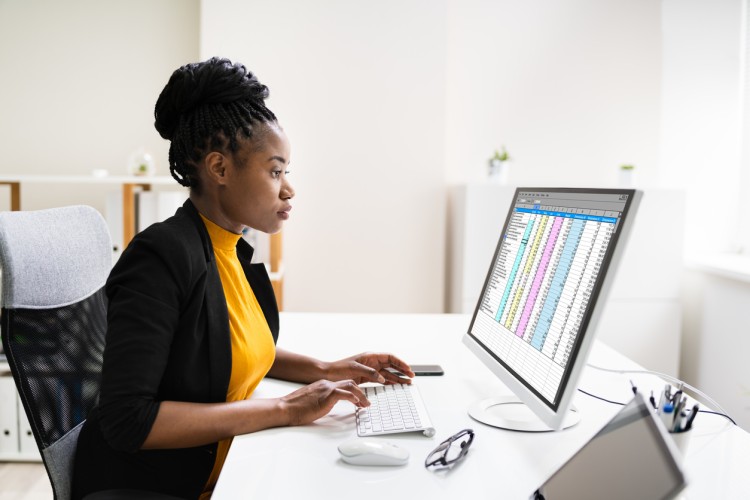
Data wrangling is an essential part of carving meaningful insights out of massive amounts of data, and data wranglers have strong job prospects as companies large and small increasingly use data analysis to guide business decisions. The exciting field of big data cannot function without professionals who have the right training and expertise to prepare data for analysis processes.
Students interested in pursuing a career in the growing data analysis realm should explore what data wrangling is all about, why it’s an important field, and the education and experience needed to succeed in a data wrangling career. Completing an advanced online certificate or degree program can be an essential step in gaining the in-demand skills needed to pursue advanced big data positions such as data wrangler. Individuals may decide to pursue a comprehensive master’s degree in data science or data analytics, or they may choose a quicker, more condensed program such as a post-bachelor’s certificate in big data.
Data Wrangling Defined
Data wrangling, sometimes known as data munging, is the process of manually cleansing raw data prior to analysis. Some of the most common tools and programming languages used in data wrangling include Microsoft Excel, SQL, Python, and Pandas. Data wranglers manually identify data gaps such as missing cells in spreadsheets, delete or fill in incomplete cells, and identify and delete irrelevant data outliers. If data isn’t cleansed properly, analytics results are often less clear and accurate.
Data Wrangling Steps
Data wrangling involves numerous steps: discovering, structuring, cleansing, enriching, validating, and publishing.
- Discovering data: In the discovery phase, data wranglers work to learn what their data is about and how it can be further explored and analyzed. For example, does it include shoppers’ browsing and purchasing history, or a detailed history of social media users’ past likes?
- Structuring data: Data comes in all shapes and sizes. Data structuring (or restructuring) is the process of merging numerous datasets into a singular unified format.
- Cleansing data: Datasets are often incomplete. Consequently, data is manually cleansed before it is analyzed. This may involve deleting and replacing inaccurate or corrupt records.
- Enriching raw data: Data enrichment involves enhancing existing data with relevant data from other sources. For example, online shopping platforms can link shoppers’ purchase history with their IP addresses to make more targeted, specific product recommendations.
- Validating data: Data validation ensures the accuracy of data before it’s analyzed. For example, if a company wants to analyze past fourth-quarter inventory levels against prior fourth-quarter purchase orders, data validation will verify that information from other quarters isn’t included in the dataset.
- Publishing data: This is the final step in data wrangling. Data publishing is when a cleansed, organized dataset is sent for analysis.
Work Settings for Data Wranglers and Data Analysts
Most professionals in this field work full time, and last-minute requests and time-sensitive projects may require overtime hours. In some instances, workers may need to travel to attend conferences and seminars. The U.S. Bureau of Labor Statistics (BLS) reports that as of 2020, the largest employers of computer and information research scientists, the closest employment category to data wranglers and data analysts, were the federal government, research and development firms, and computer services providers.
Data Analytics Job Growth Projections
The BLS projects overall employment in the computer and information research scientist field to grow by 22% between 2020 and 2030, far faster than labor market growth as a whole (8%). The BLS notes that rapid increases in data collection by companies, along with the demand for new and better technology systems, will drive growth in the field.
Skills Data Wrangling Specialists Need to Succeed
Students interested in learning more about what data wrangling is will find that aspiring data wranglers need various skills and competencies. Examples include analytical, programming, logical thinking, and problem-solving skills.
- Analytical skills: Data wranglers must review and evaluate various datasets to identify relevant information. Strong analytical skills are required to evaluate large, unstructured data sources.
- Programming skills: Data wranglers need to have a strong background in computer programming languages such as SQL and Python. These languages help analysts clean datasets using automated processes.
- Logical thinking skills: Unstructured data comes in various sizes and forms. Data wranglers with refined logical thinking skills understand how to combine and restructure multiple datasets into an analysis-friendly format.
- Problem-solving skills: If data analytics is like solving a puzzle, data wrangling is the process of restructuring wood fibers, paint, and glue to create puzzle pieces. Problem-solving skills help data wranglers decide which datasets are relevant and determine the transformations to perform before the data is published.
Earn a Certificate in Big Data and Prepare to Advance Your Career
Careers in big data, including data wrangling and data analytics, are growing rapidly. Students interested in upskilling or advancing their data analytics skills will find that online degree programs such as Maryville University’s online Master’s in Data Science or Master of Science in Data Analytics can prepare them for the next phase of their careers. Individuals who are interested in a shorter path toward an advanced data analytics career can explore Maryville’s online Post-Baccalaureate Certificate in Big Data, which provides students with access to commonly used data wrangling tools and techniques.
Discover how Maryville can help you expand your understanding of big data analytics.
Recommended Reading
4 Benefits of Data Analytics in Healthcare
5 Winning Data-Driven Marketing Strategies
Making a Positive Impact: How Data Science Is Being Used for Social Good
Sources
Altair, “What Is Data Wrangling?”
Astera, “What Is Data Wrangling and Why Do You Need It?”
Express Analytics, “What Is Data Wrangling? What Are the Steps in Data Wrangling?”
Hevo, “Data Wrangling in 6 Steps: An Analyst’s Guide For Creating Useful Data”
SolveXia, “What Is Data Wrangling and Why It’s So Important”
Trifacta, “How to Clean Data: Cleaning, Cleansing & Scrubbing”
Trifacta, “What Is Data Munging?”
Trifacta, “What Is Data Wrangling?”
U.S. Bureau of Labor Statistics, Computer and Information Research Scientists