Understanding How AI Works: A Comprehensive Guide
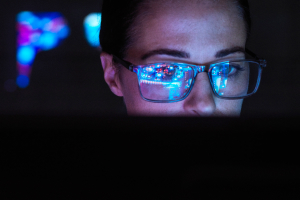
Artificial Intelligence (AI) has become indispensable in our daily lives, revolutionizing industries and reshaping our interaction with technology. It permeates nearly every facet of modern technology, from virtual assistants like Siri and Alexa to autonomous vehicles navigating our streets and airspace.
As AI continues to shape our world profoundly, it’s essential to understand the fundamentals behind this transformative technology. Whether you’re a seasoned professional in the field or a curious beginner, this guide will provide a comprehensive overview of how AI works, breaking down complex concepts into digestible insights.
Fundamentals of Artificial Intelligence
Artificial Intelligence (AI) is a field of computer science dedicated to creating systems that can perform tasks that typically require human intelligence. These tasks include learning, problem-solving, understanding natural language, and perception. Within AI, several distinct subsets have emerged, each contributing unique dimensions to its functionality:
- Machine learning (ML): At its core, machine learning mirrors the cognitive training process in humans. Computers learn from data and examples, gradually enhancing their performance without explicit programming. This empowers machines with the ability to learn and adapt autonomously.
- Deep learning: Inspired by the complex neural networks of the human brain, deep learning involves systems with multiple layers of interconnected “neurons.” This sophisticated approach enables computers to discern intricate patterns in data, facilitating tasks such as image and speech recognition with remarkable precision.
- Natural language processing (NLP): NLP bridges the gap between human language and machine comprehension. Through NLP, computers can interpret and generate human language, powering applications like chatbots, translation services and sentiment analysis.
- Computer vision: Computer vision technologies help systems perceive and comprehend visual information akin to human vision. This enables computers to identify objects, interpret scenes and navigate environments, revolutionizing industries such as autonomous driving and healthcare imaging.
While these AI disciplines may sound complex, their real-world applications are both profound and accessible, enriching our lives with newfound efficiency and innovation. Embracing these technologies opens doors to a future where human-machine collaboration yields unprecedented possibilities, enhancing our experiences and capabilities across diverse domains.
How AI works: The steps of Artificial Intelligence
Let’s explore how AI works through the lens of personalized streaming recommendations. Picture logging into your favorite streaming platform and instantly encountering a tailored selection of shows and movies. This seamless experience is powered by a sophisticated AI system that analyzes vast data sets to deliver personalized recommendations.
1. Data Collection: Data collection is the process of gathering relevant information from various sources to support the functioning of an AI system. It involves systematically acquiring data points for the AI system to learn patterns, make predictions or perform other tasks.
Imagine your favorite streaming platform gathering information about your viewing habits, preferred genres, directors and networks. This data forms the bedrock of personalized recommendations, enabling the AI system to understand your preferences. Some platforms even employ systems to help collect more relevant data, such as rating or like/dislike systems.
2. Data Preprocessing: After gathering the data, it undergoes preprocessing, which involves cleaning, transforming and standardizing it for consistency across datasets. For personalized streaming recommendations, this includes removing duplicates and addressing missing values through techniques like imputation.
3. Feature extraction: This process involves identifying relevant attributes or features from the preprocessed data to empower AI systems to generate personalized recommendations. Features such as genre preferences, viewing history and device usage serve as inputs for the recommendation algorithm.
4. Model selection: This step entails choosing the most suitable machine learning algorithm for generating personalized recommendations on streaming platforms. Approaches like collaborative filtering, content-based filtering and hybrid methods can enhance user experience.
Collaborative filtering analyzes user behavior to recommend content similar to what similar users have enjoyed, while content-based filtering focuses on content attributes. Hybrid approaches combine both methods for improved recommendation accuracy and coverage. By selecting a suitable model, streaming platforms can deliver accurate and relevant suggestions to enhance user experience.
5. Training: During training, the model learns from past user data and the features identified, such as users’ viewing habits and preferences. It seeks patterns in this data, like which genres tend to be watched together, adjusting itself accordingly. The model improves its ability to predict what users might enjoy through this process.
6. Evaluation: In the evaluation phase, analysts use separate data to assess the model’s performance by comparing its predictions to actual user behavior. They investigate any discrepancies to identify areas for improvement and ensure the accuracy and fairness of the recommendations provided.
7. Fine-tuning: Following evaluation, adjustments may be made to further enhance the model’s performance. This involves tweaking parameters such as learning rates or feature weights based on user feedback and evaluation results. Additionally, incorporating feedback mechanisms, such as user ratings or explicit feedback, can help refine the model’s predictions over time, ensuring that recommendations remain relevant and engaging for users.
8. Deployment: Once deemed adequate, the model is deployed into the streaming platform, where it begins generating recommendations for users. This involves seamless integration into the streaming platform, ensuring scalability, reliability and security.
9. Monitoring and maintenance: After deployment, the model is continuously monitored to ensure it continues to perform effectively. Adjustments to the model can address issues, and regular updates ensure the model adapts to evolving user preferences and content offerings.
Embrace innovation with advanced AI training
Artificial Intelligence has evolved from a theoretical concept to a transformative force driving innovation across industries. By understanding the fundamentals of AI, from data to deployment, we gain insight into its inner workings and profound impact on our lives. Embracing these technologies opens doors to a future where human-machine collaboration yields unprecedented possibilities, enhancing our experiences and capabilities.
As the world of AI continues to grow, the need for dedicated and trained professionals working in this space will also grow. Explore the opportunities through Maryville University’s online Master of Science in Artificial Intelligence and AI certificate programs. Whether you’re looking to advance your career or explore more about how AI works, Maryville University provides the tools and resources you need to succeed in this dynamic and rewarding field.
Recommended reading: